Top algo trading strategies
Top Algo Trading Strategies:
Scalping based algorithmic trading to profit from short-term price fluctuations
Momentum trading - to identify strong recent trends, and enter positions
Moving Average to Minutes (TMA-M) based algorithmic trading
Trend following algorithmic trading to profit from riding the prevailing market trends
Risk-on/risk-off - two distinct strategies that guide investment decisions based on the prevailing market sentiment
In this post, we delve into the world of algorithmic trading strategies, shedding light on their key components and benefits. We explore popular approaches like trend following, mean reversion, and pairs trading, explaining how they capitalize on market dynamics. Readers will discover the power of backtesting and optimization in refining their strategies, while emphasizing the critical role of risk management. Whether a beginner or seasoned trader, this post offers actionable insights to harness the potential of algo trading and navigate the markets with confidence.
What is algorithmic trading?
Algorithmic-based trading, also known as algorithmic trading or algo trading, is a sophisticated approach where trading decisions are executed automatically by computer algorithms or automated trading software.
These algorithms play a crucial role by providing the tools and infrastructure to implement, test, and execute trading strategies efficiently and effectively. These algorithms are designed to analyze vast amounts of data, detect patterns, and execute trades at high speeds without direct human intervention.
Best algo trading strategies
Provided below is a brief overview of 10 of the best algo trading strategies.
Scalping
Scalping based algorithmic trading is a high-speed strategy that aims to profit from short-term price fluctuations. Using computer algorithms, scalpers execute a large number of trades within seconds or minutes, seeking to capitalize on tiny price differentials. One way to enhance high speed trading is to utilize an automated trading robot.
These algorithms analyze tick data and real-time market feeds, identifying patterns or arbitrage opportunities to make rapid trading decisions. Scalping is often associated with high-frequency trading (HFT), as it requires low-latency connections and specialized infrastructure to gain a competitive edge. Some scalping strategies also act as liquidity providers, narrowing bid-ask spreads and enhancing market efficiency.
Strict risk management rules are essential for scalping algorithms to avoid significant losses. Successful scalping algorithms thrive in highly liquid markets with sufficient volatility, where short-term price movements are prevalent.
Momentum
Momentum based algorithmic trading capitalizes on the continuation of existing price trends in financial markets. The core principle behind this approach is that assets that have shown strong recent price movements, either up or down, are likely to continue moving in the same direction for a certain period. Momentum algorithms are designed to identify these trends, enter positions in the direction of the momentum, and exit when the trend starts to lose strength.
To implement momentum based algorithmic trading, historical price data is analyzed to identify assets with significant price changes over a specified time frame. Common Momentum trading technical indicators such as moving averages, Relative Strength Index (RSI), and MACD (Moving Average Convergence Divergence) are often used to identify momentum signals.
When an asset exhibits a strong momentum signal, the algorithm triggers a trade in the direction of the trend.
Taking Moving Average to Minutes
Taking Moving Average to Minutes (TMA-M) based algorithmic trading adapts the traditional Moving Average (MA) strategy to operate on shorter time frames, specifically minutes. The Moving Average is a technical indicator that smooths out price data over a specific period, revealing trends and potential entry/exit points. Instead of using daily or hourly data, the algorithm utilizes minute-by-minute price data to calculate the Moving Average.
The TMA-M algorithm tracks short-term price trends within a single trading day by continuously updating the Moving Average based on minute intervals. By doing so, it provides a more granular and real-time view of the market, allowing for faster responses to price movements.
Traders can implement different variations of the TMA-M strategy, such as using a combination of shorter and longer time frame Moving Averages to generate crossover signals or employing dynamic thresholds to trigger trades. TMA-M can be applied to various financial instruments, including stocks, Forex pairs, and cryptocurrencies. For example, TMA-M algo trading allows auto traders to automate certain standard Forex trading strategies.
Trend Following
Trend following based algorithmic trading is a popular strategy that seeks to profit from identifying and riding the prevailing market trends. The core concept behind this approach is to enter long positions during uptrends (rising prices) and short positions during downtrends (falling prices). The algorithms used in trend following analyze historical price data to identify trends, typically by employing technical indicators like Moving Averages, Average True Range (ATR), or the Directional Movement Index (DMI).
When a trend is detected, the algorithm triggers a trade in the direction of the trend and aims to stay in the position until the trend shows signs of weakening or reversing. Trend following algorithms may use trailing stop-loss orders to protect profits and exit trades when the trend loses momentum.
Trend following based algorithmic trading can be applied to a wide range of assets, including stocks, commodities, currencies, and futures. It can be effective in both short-term and long-term trading horizons, depending on the time frame used to detect trends.
Risk-on/risk-off
Risk-on and risk-off based algorithmic trading are two distinct strategies that guide investment decisions based on the prevailing market sentiment and perceived levels of risk in financial markets.
Risk-on trading refers to a market environment where investors exhibit a higher appetite for risk, leading them to invest in assets with higher expected returns, such as equities, high-yield bonds, and commodities. In risk-on periods, algorithmic trading strategies may focus on identifying assets that tend to perform well when investors are optimistic about economic growth and less concerned about potential losses. These algorithms may use technical indicators or sentiment analysis to gauge market sentiment and identify opportunities in riskier assets.
On the other hand, risk-off trading occurs when investors become more risk-averse and seek safer assets, such as government bonds, gold, or other defensive securities. During risk-off periods, algorithmic trading strategies may aim to capitalize on market downturns or volatility by short-selling risky assets or investing in safe-haven assets. These algorithms may rely on indicators like volatility indices or economic data releases to identify shifts in market sentiment and adjust positions accordingly.
Inverse Volatility
Inverse volatility based algorithmic trading capitalizes on the inverse relationship between volatility and asset prices. Volatility refers to the degree of fluctuation in an asset's price over a specific period. Inverse volatility algo trading algorithms take advantage of the fact that when volatility rises, asset prices tend to fall, and vice versa.
The algorithm identifies periods of elevated volatility and executes trades to profit from potential price rebounds when volatility subsides. Typically, these algorithms employ exchange-traded products (ETPs) such as inverse volatility ETFs or volatility futures to achieve the desired inverse exposure.
Inverse volatility strategies are often implemented by sophisticated algo traders and hedge funds seeking to benefit from mean-reverting behavior in the market. These algorithms may use statistical analysis and technical indicators to identify potential turning points in volatility, and subsequently, in asset prices.
Black Swan Catchers
Black Swan catchers based algorithmic trading mitigates the risks associated with rare and extreme events, known as Black Swans, that have a severe impact on financial markets. Black Swan events are unexpected and difficult to predict using traditional models, making them a significant challenge for auto traders.
The algorithmic trading approach aims to detect early warning signs of potential Black Swan events by monitoring various market indicators, macroeconomic factors, and other relevant data sources. These algorithms may incorporate machine learning, artificial intelligence, and statistical methods to identify patterns or anomalies that could indicate the onset of a Black Swan event.
When the algorithm detects heightened risk or unusual market behavior that may precede a Black Swan event, it can trigger defensive measures, such as reducing exposure to risky assets, hedging positions, or even exiting the market entirely. By taking proactive steps to protect the portfolio from extreme market disruptions, Black Swan catchers aim to minimize potential losses and preserve capital during turbulent times.
Simple Moving Average Crossover
Simple Moving Average (SMA) crossover based algo trading strategies leverage the crossover of two different SMAs to generate buy and sell signals in financial markets. The SMA is a technical indicator that calculates the average price of an asset over a specified period, typically using closing prices. Auto traders employ two SMAs with different timeframes, commonly a short-term SMA (e.g., 50-day) and a long-term SMA (e.g., 200-day).
When the short-term SMA crosses above the long-term SMA, it generates a "golden cross" signal, indicating a potential uptrend and a buy signal. Conversely, when the short-term SMA crosses below the long-term SMA, it generates a "death cross" signal, indicating a potential downtrend and a sell signal.
Mean Reversion
Mean reversion based algo trading relies on the belief that asset prices tend to revert to their historical average or mean over time. The core concept behind this approach is that when prices deviate significantly from their average, they are likely to reverse direction and move back towards the mean.
Algorithmic mean reversion strategies typically use statistical analysis and technical indicators to identify assets that are overbought or oversold. When an asset's price deviates too far from its historical average, the algorithm triggers a trade in the opposite direction, anticipating a reversion towards the mean.
The mean reversion strategy is commonly applied to pairs trading, where two related assets, such as two stocks in the same industry, are traded simultaneously. The algorithm looks for deviations in the price ratio between the two assets and takes long and short positions to capitalize on the expected convergence back to their historical relationship.
Pairs Trading
Pairs trading based algorithmic trading capitalizes on the relative price movements of two correlated assets. The core principle behind this approach is that assets with a historically strong correlation tend to move together over time. However, in certain instances, temporary price divergences may occur due to market inefficiencies or other factors. Pairs trading algorithms aim to identify such deviations and take advantage of them by establishing a long position in the underperforming asset and a short position in the outperforming asset.
To implement pairs trading, the trading algorithms select two assets with a strong historical correlation and calculate a statistical measure of their relationship, often using techniques like cointegration or the distance between their prices. When the price spread between the two assets widens beyond a defined threshold, the algorithm executes the long-short positions, anticipating the spread to revert back to its historical mean.
Best brokers for automated trading
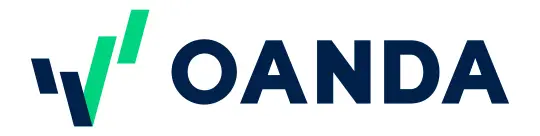
Algo trading: why should I choose it?
Here's a summary of the primary algo trading benefits.
Trading algorithms can analyze vast amounts of data and execute trades at lightning-fast speeds, enabling quick reactions to market opportunities and reducing the risk of missing profitable trades.
Algorithmic trading removes human emotions from the decision-making process, preventing impulsive and irrational trading decisions driven by fear or greed.
Algorithms can be thoroughly backtested and optimized using historical data, helping traders fine-tune their strategies for better performance and risk management.
Algo trading allows traders to operate across various markets and assets simultaneously, such as futures, stocks, forex etc., spreading risk and reducing exposure to individual assets.
These trading algorithms can execute trades with precise parameters, ensuring orders are placed at specific prices and minimizing slippage.
Trading algorithms follow predefined rules consistently, helping traders maintain discipline and avoid impulsive trading decisions.
Automated systems can monitor and trade markets around the clock, taking advantage of global opportunities even when the trader is unavailable.
Algo trading incorporates sophisticated risk management techniques, including stop-loss orders and position sizing, to control potential losses.
Algorithmic trading reduces the chance of human errors in trade execution, helping maintain a high level of accuracy.
For traders engaging in HFT, algorithmic trading is essential to execute a large number of trades within extremely short time frames.
Algorithms can implement complex trading strategies that would be difficult or impossible to execute manually.
How to create an algo trading strategy?
Creating an algorithmic trading strategy involves a systematic approach and careful consideration of various factors. Below is a detailed step-by-step guide to help you create your own algo trading strategy.
Clearly outline your trading objectives, including your desired risk-reward profile, time horizon, and target markets or assets you wish to trade.
Determine the type of strategy you want to implement, such as trend following, mean reversion, pairs trading, or others, based on your objectives and market analysis.
Conduct thorough research on your chosen market or assets. Analyze historical price data, identify relevant technical indicators, and look for patterns or correlations that could form the basis of your strategy.
Develop specific rules for entering and exiting trades based on your research. Define the conditions under which your strategy will generate buy or sell signals.
Use historical data to backtest your trading strategy. This step is crucial to evaluate its performance and potential profitability in different market conditions.
Fine-tune your strategy by adjusting parameters and variables based on the backtesting results. Strive for a balance between profitability and risk management.
Integrate risk management techniques, such as stop-loss orders, position sizing, and diversification, to protect your capital and manage potential losses.
Choose a trading platform or API that supports algorithmic trading. Or, if you are interested in Forex trading, select a Forex algo trading platform that suits your needs.
Test your algorithm in a simulated environment or with paper trading to ensure it functions as intended without risking real money.
Regularly monitor your strategy's performance and adjust it as needed to adapt to changing market conditions.
Ensure that your algorithmic trading complies with all relevant laws and regulations in the jurisdictions where you operate.
Stay updated with the latest developments in algorithmic trading, markets, and technology to continuously improve and innovate your strategy.
Key tips for algo trading beginners
For algo trading beginners, it's essential to begin with straightforward strategies that are easier to understand and implement. Avoid overly complex strategies until you have gained more experience and confidence in algorithmic trading.
Always backtest your trading strategies using historical data to evaluate their performance and potential profitability. Optimization allows you to fine-tune parameters for better results, but be cautious not to overfit the strategy to past data.
Prioritize risk management to protect your capital. Use techniques like setting stop-loss orders, proper position sizing, and diversification to manage potential losses and maintain a balanced risk-reward profile.
Before trading with real money, practice with paper trading or simulated environments to test your strategy's effectiveness without risking capital. This helps identify and address potential issues before going live.
Keep yourself updated with market trends, news, and technological advancements in algorithmic trading. Markets can change rapidly, so be prepared to adapt your strategies to new conditions and continuously improve your skills as an algo trader.
Summary
Algorithmic trading strategies have revolutionized the way auto traders participate in financial markets. By leveraging data analysis, automation, and risk management techniques, algo trading opens doors to new opportunities and enhances decision-making precision. Embracing algorithmic trading empowers traders with the tools to navigate the complexities of modern markets and ultimately unlock their full trading potential. So, embark on this exciting journey, refine your strategies, and embrace the world of algo trading to stay at the forefront of the ever-evolving trading landscape.
FAQ
What is the best strategy for algo trading?
The best strategy for trading depends on individual preferences, risk tolerance, and market conditions. Some popular strategies include trend following, mean reversion, and pairs trading, but it's essential to thoroughly research, backtest, and optimize any strategy before implementing it in real markets.
Is algo trading profitable?
Algo trading can be profitable for those who develop effective strategies and have a thorough understanding of the markets. However, success depends on factors such as strategy design, risk management, and adaptability to changing market conditions.
Is algo trading difficult?
Algo trading can be challenging for beginners due to the need for data analysis and strategy development. However, with dedication, learning, and practice, individuals can overcome these challenges and find success in algo trading.
What is the success ratio of algo trading?
The success ratio of algo trading varies widely and depends on the effectiveness of the trading strategies used. Well-designed and optimized algorithms can have a higher success ratio, but there are no guarantees, and success ultimately depends on the trader's skills, risk management, and adaptability to market conditions.
Team that worked on the article
Thomas Wettermann is an experienced writer and a contributor to the Traders Union website. Over the last 30 years, he has written posts, articles, tutorials, and publications on several different high tech, health, and financial technologies, including FinTech, Forex trading, cryptocurrencies, metaverses, blockchain, NFTs and more. He is also an active Discord and Crypto Twitter user and content producer.
Dr. BJ Johnson is a PhD in English Language and an editor with over 15 years of experience. He earned his degree in English Language in the U.S and the UK. In 2020, Dr. Johnson joined the Traders Union team. Since then, he has created over 100 exclusive articles and edited over 300 articles of other authors.
Mirjan Hipolito is a journalist and news editor at Traders Union. She is an expert crypto writer with five years of experience in the financial markets. Her specialties are daily market news, price predictions, and Initial Coin Offerings (ICO).